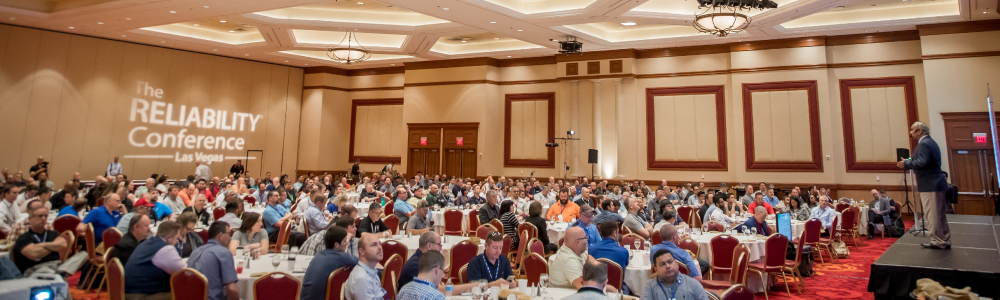
TOP FIVE TAKEAWAYS FROM THE 2018 RELIABILITY CONFERENCE
MAY 2, 2018 - DAVID SIEGEL, CTO
Predictronics Chief Technology Officer David Siegel attended The Reliability Conference (TRC) April 23-28 in Las Vegas, where he discussed predictive monitoring technologies, Artificial Intelligence (AI) and the Internet of Things (IoT).
During the conference, Dr. Siegel also presented a case study in collaboration with air conditioning manufacturer Daikin on the topic of predictive monitoring for early detection and diagnosis of air conditioning units.
Below are Dr. Siegel’s top five conference takeaways:1. Organizational Change Might be the Biggest Barrier
A common question addressed during the conference was how to overcome organizational and management challenges when it comes to the implementation of maintenance and reliability practices. It appears that organizations with solid maintenance and reliability practices will be the first to adopt new AI and IoT technologies. That is because companies must learn how to walk before they can run. It becomes difficult to move toward a condition-based or predictive maintenance approach without first experiencing some level of organizational change.
2. The combination of AI /IoT with Domain Expertise
Technologies that are able to recognize and learn data patterns to ultimately understand the health condition of assets have been demonstrated and deployed in many applications. Nevertheless, AI and machine learning only work if the problem is well defined and the domain knowledge is incorporated to bound the data-driven models. The most successful case studies presented at the conference were ones that included both machine learning and domain expertise. 3. Interest from a Variety of Industries
The conference included representatives from various industries, including power generation, oil and gas, mining, transportation and manufacturing. That means asset management and reliability practices are important to multiple industrial segments and often represent savings in both downtime and dollars that make a compelling business case.
4. The Right Data at the Right Quality
The right data at the right quality is necessary to exctract value. Asset health visualization and machine failure predictions can be automated with AI and machine learning platforms, but only if the available data is suitable for this objective. In many cases, building a predictive monitoring system is an iterative process. If one is not getting results, it could be that the data quality is simply not good enough.5. Focusing on the Right Problem
Finding the right problem requires domain expertise, criticality analysis and proper usage of maintenance records to determine which equipment or processes should be the target of a predictive monitoring system. The problem selection should also consider the frequency of failure, the feasibility for monitoring machine health and whether or not a failure is likely to occur during the pilot project. In most cases, a successful pilot starts with the selection of a problem that is both valuable and technically feasible. Not selecting the right problem limits the project’s likelihood of success.
For more information and weekly updates, follow Predictronics:
Know What Happens Next with PDX!
TRY IT TODAY