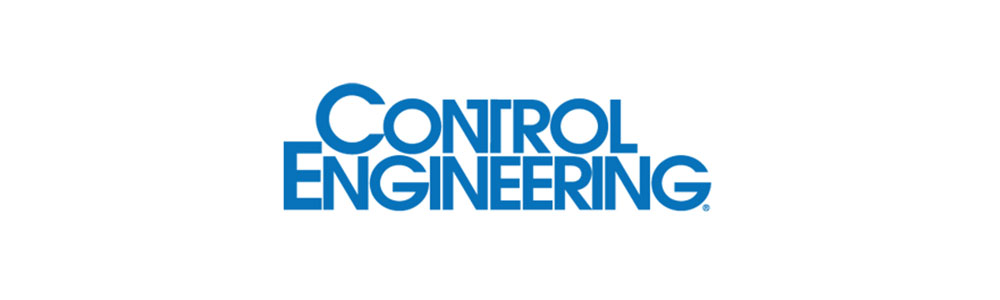
PREDICTRONICS CTO TAKEAWAYS FROM THE CONTROL ENGINEERING MAGAZINE IIOT SERIES WEBINAR
December 3, 2020
Predictronics CTO Dr. David Siegel had the opportunity to co-host a webinar for the Control Engineering Magazine IIoT Edge/Cloud Webcast Series entitled Machine Learning and Pattern Recognition with our sales partner and CEO of IoTco, Dr. Mohamed Abuali.
The goal of this webinar was to showcase the value of digital transformation, powered by IIoT and predictive analytics, through predictive quality solutions for process optimization and ROI, as well as demonstrate how machine learning and pattern recognition can be incorporated into the development of these solutions.
During the webinar, Dr. Siegel presented three successful Predictronics case studies for predictive quality, including process optimization for CPG manufacturing, anomaly detection for faulty automotive products, and predictive quality for automotive die casting.
Dr. Siegel demonstrated the methodology and workflow for these predictive quality solutions, which involves pre-processing steps, feature engineering, and machine learning to relate process conditions to potential issues in the process and quality. If applied correctly to a rich and accurate data set, this template can help improve product quality, reduce scrap, avoid wasted production time, and increase productivity.
Below are Dr. Siegel’s three major takeaways from the webinar:
The Role of Domain Experts
One audience member webinar asked if process and manufacturing engineers help data scientists with the configuration or use of these types of AI-based predictive quality solutions.
The best and most accurate solutions combine data-driven methods with domain knowledge and insight. Therefore, engineers can play a key role in the configuration of these solutions for the manufacturing process, as they have a wealth of helpful experience and expertise within their specific industry. Domain experts can aide in providing key context, as well as in identifying critical process parameters, determining thresholds, providing quality metrics, giving feedback on visualizations, and guiding deployment, among other tasks.
Supervised and Unsupervised Learning for Predictive Quality
Another participant questioned whether unsupervised or supervised machine learning is best suited for predictive quality solutions.
Unlike predictive maintenance solutions, which utilize unsupervised learning due to the rarity of failures, predictive quality solutions can used both supervised and unsupervised models. No matter the type of model, it is important to provide variable and feature contribution information, so the root-causes of the process and quality issues can be investigated.
Optimal Design Strategies for Predictive Solutions
This webinar also included a discussion about the value of process and quality data in informing design.
Predictive solutions can not only provide impact through improved product quality, but they can also provide critical feedback for product and process design. Persistent product quality issues can be measured and analyzed to determine what is contributing the most to those issues, be it a specific parameter or a product quality metric being out of spec.
Such consistent issues can serve as the basis for improvements in process design or product design, if another root cause cannot be identified. Establishing a standardized approach helps to determine which issues can be addressed with predictive quality and which can be tackled through design changes. This presents another opportunity to leverage domain knowledge, in terms of how to best translate analysis results into design tasks.
Overall, the webinar sparked a great discussion on the current state of predictive quality solutions, their business value, and future areas of work needed.
For more information and weekly updates, follow Predictronics:
Know What Happens Next with PDX!
TRY IT TODAY