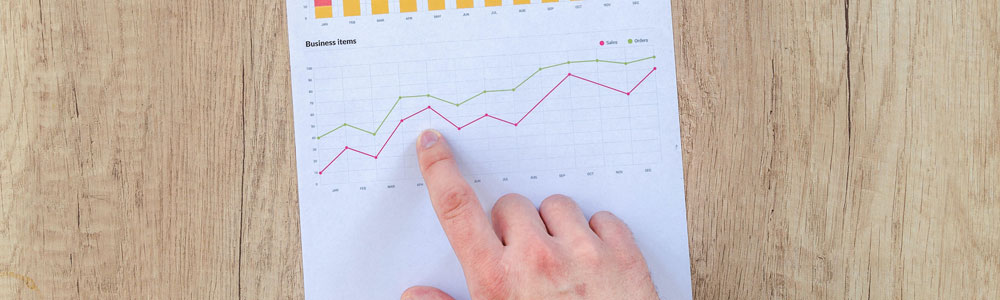
CUSTOMER SUCCESS SERIES PART I: TOP PRE-ENGAGEMENT QUESTIONS ASKED BY PREDICTRONICS CUSTOMERS
AUGUST 9, 2023
In order to achieve the greatest success with our clients, it is essential that those seeking digital transformation have an understanding of what they need and the services Predictronics provides, our team background and how those skills translate into customer ROI, as well as tips and best practices for adopting and deploying predictive analytics for their industrial assets. This blog series aims to address these many quandaries, with knowledge the Predictronics team has acquire from our years of experience, in order to help others to make the right decisions regarding their data and asset management.
When planning for predictive, and later developing and managing a solution, Predictronics customers frequently have the same common questions for our team concerning their data availability and quality, the role of their own team members, the functionality of our software, the proof of value process, and more. In this first installment of our series, we have gathered a select handful of the most popular pre-engagement questions asked by customers and our responses, regarding their needs, the solution building process, and our approach. Our expertise will help guide you through your own preparations.
How do I select what assets to monitor for a faster ROI?
When determining what assets to monitor, you must see that it makes both economic sense for your company, as well as whether it is feasible based on the data you have, the ability to set up the system, and more.
Key assets can be identified through criticality analysis. Criticality analysis is the systemic method of evaluating risks and ranking assets. This approach considers the frequency of failures, the cost or downtime associated with the failures, and the cost of the asset. Criticality analysis is typically done top-down. First, the key assets impacting overall production, efficiency, ROI, and/or quality are identified and assessed. Next, analysis is performed at a component level to better understand which components are the most critical within the asset. The list of critical assets and components then undergo some feasibility considerations to establish whether [a] the appropriate machine data can be retrieved via communication protocols, [b] add-on sensors can be safely and appropriately installed in optimized locations to get the best quality of data, and [c] the critical assets to be monitored are 'old enough' to expect a failure during project implementation. The components selected are then monitored using a predictive maintenance solution.
How long is a proof-of-value engagement?
A typical proof of value engagement lasts three to six months, focusing on the configuration and development of a predictive maintenance predictive health monitoring, and/or predictive product quality solution for a subset of critical assets. The success and time frame of this project relies solely on the accuracy and timeliness of the data insights and predictions.
Learn more here about Predictronics’ specific proven PoV process for working with new clients in the pilot phase that has resulted in numerous successful engagements.
What human resources are needed from the customer side to engage in a PoV?
Obtaining buy-in, from stakeholders and project managers to solution end-users and IT experts, is essential. Shifting the culture within an organization can be one of the keys to achieving digital transformation and, specifically, shifting from current traditional approaches to those that incorporate machine learning and AI for predictive solutions.
Our engineers and experts have valuable insights about our machines and processes. How are these insights incorporated into the deployed solution?
Domain expertise is highly valuable and can be incorporated into many aspects of a solution. Your team can add context during the data acquisition process, such as pinpointing what data to collect or ignore and identifying interesting segments in the data. This will help optimize data collection. After initial analysis of the data, our team and the customer collectively review the parameters/features that Predictronics thinks are most significant to the application before the model is finalized. Feedback from your team helps to improve this model accuracy and development. When a failure event is predicted by the deployed model, our team will revisit the model with your domain experts to see if this degradation alert is as expected or if the model needs updating. This helps us prevent false alarms and fine-tune our algorithm for the best data results.
If I already have some data, how do I gauge whether it is useful for predictive maintenance and/or quality?
OR
If I am already collecting the necessary data, how is this data then ingested?
Predictive Maintenance - Depending on the application and the type of failure you are trying to predict a good starting point is to gauge if the data available Is physically relevant to the pain point. If that's already the case, then our Predictronics team can dive deeper into the quality of the data itself.
Predictive Quality - A common approach for this application is to predict part quality based on process or recipe parameters. If the relevant parameters are captured on a part basis, you are on the right track.
In both cases, the manner of data ingestion is dependent on the source. Predictronics can read data directly from databases or use standard industrial protocols, like OPC UA and MTConnect. In some cases, we may recommend sensors and hardware for additional data collection.
What data do I need to provide? Do I need sensors?
The type of data needed depends on the individual asset and application, Sensors are typically required if a valuable source of information has not been captured or the quality of the data collected is not at the level necessary to achieve a successful predictive solution with the most accurate insights. To give an example, if you are trying to monitor spindle health on a CNC, Predictronics would recommend utilizing accelerometers to collect vibration data.
Once you have decided to pursue a predictive solution, and have selected an appropriate target asset or process, other considerations come to mind when deploying and maintaining that solution.
In part two of our series, we will present the most frequently asked questions from our customers concerning the technical aspects of this process after an engagement has begun, as well as provide answers to those questions.
For more information and weekly updates, follow Predictronics:
Know What Happens Next with PDX!
TRY IT TODAY